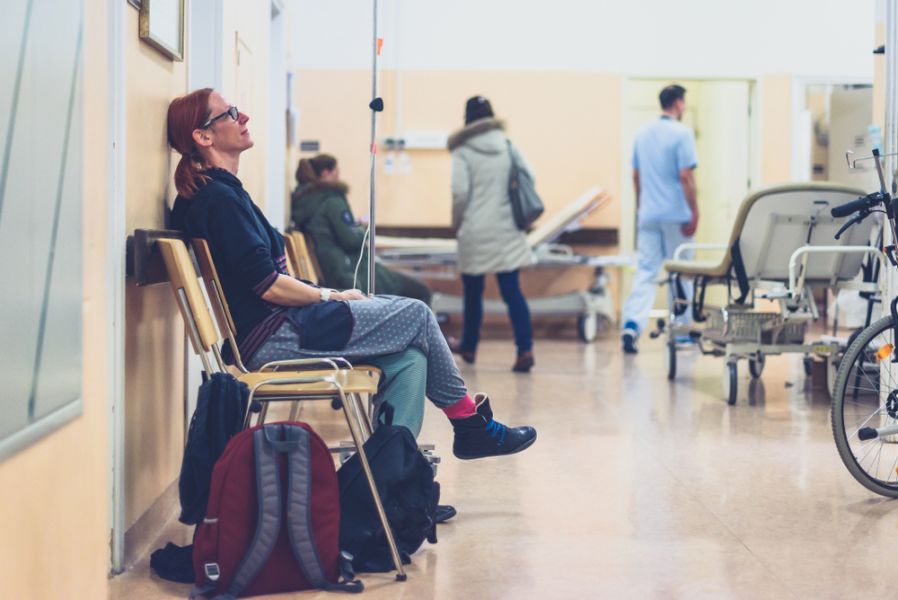
New research from the Australian Institute of Tropical Health and Medicine is using machine learning algorithms to improve health outcomes for patients waiting in public hospital Emergency Departments.
AITHM Health Economist and Data Scientist Dr Anton Pak said current systems of forecasting patient waiting times did not account for the dynamic and complex nature of hospital Emergency Departments.
However, his research had implemented machine learning algorithms to deliver greater modelling accuracy on waiting time forecasts.
Dr Pak said the projected impact of the research would be in the eventual development of a public interface, which would give people access to ED waiting times in close to real-time.
“From a patient’s perspective, the knowledge may reduce anxiety and uncertainty about waiting times and improve satisfaction. It also has the potential to assist clinicians and nurses in how they estimate demand for care and to calibrate workflow," Dr Pak said.
The two-year observational study was conducted under the guidance of University of Queensland (UQ) Economics Professor Brenda Gannon, in collaboration with Brisbane's Princess Alexandra Hospital’s (PAH) Deputy Director of Emergency Medicine Dr Andrew Staib.
Dr Pak and colleagues from UQ and PAH scrutinised the movements of up to 120,000 ED patients using machine learning algorithms, to look at a large set of real-time patient information to determine their actual wait time.
The partnership facilitated the gathering of administrative data from the Hospital's ED including the patient journey from arrival to departure, over a two-year period between January 2016, and December 2017.
Dr Pak said once finalised, the system could be rolled out across Emergency Department networks in all hospitals, where patients could have access to view waiting times before deciding to go to a particular hospital.
Dr Pak said the research paper entitled: "Predicting Waiting Time-To-Treatment for Emergency Department Patients" and published in the International Journal of Medical Informatics, has shown this method to be more accurate at predicting wait times than what is currently available.
“Current systems of reporting ED waiting times to patients, are limited, and have largely included rolling average estimates or median historical waiting times,” Dr Pak said.
By implementing ML algorithms and using a large set of queueing and service flow variables, we were able to showed evidence of the improvement in waiting time predictions for low acuity ED patients assigned to the waiting room.
In addition to the mean squared prediction error (MSPE) and mean absolute prediction error (MAPE), we advocate to use the percentage of under-predicted observations.
The use of ML algorithms is motivated by their advantages in exploring data connections in flexible ways, identifying relevant predictors, and preventing overfitting of the data.